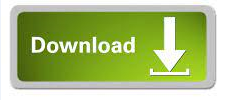
There are two inherent problems on using multiple comparisons, first is the false positive result that is higher probability of detecting non-existing positive outcome, which is a procedural problem (type I error). Failure to use appropriate statistical methods weakens conclusions (Curran-Everett, 2000). Multiple comparisons means testing more than one hypothesis, in other words it is comparing two study groups for more than one output (outcome). Testing one hypothesis (effect of a drug A to control hypertension) is primary analysis, occasionally, researchers use data obtained from the study population to examine multiple outcome variables (secondary analysis). Testing the null hypothesis serves to guard against unjustifiable conclusions. Therefore, in data analysis, it is essential to identify the variables as input, output, or confounding (Campbell, 2006).
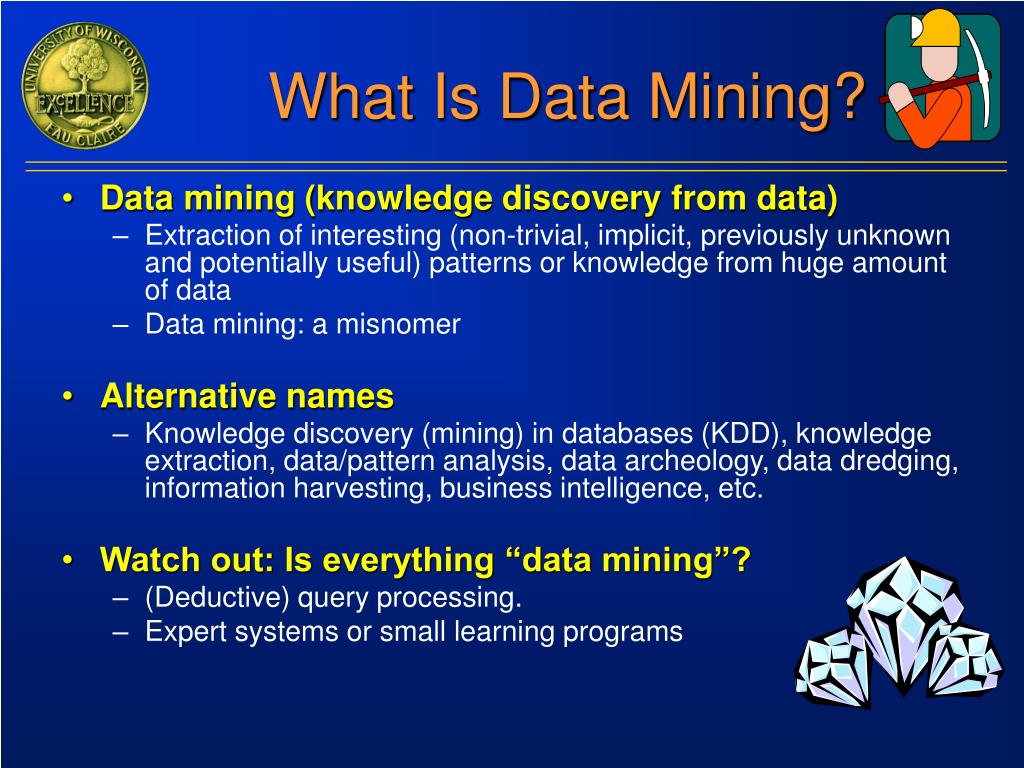
Confounding (confusing) factors may shadow either cases, in the previous example, age or gender can be confounding factors. As an example testing the link between obesity and diabetes can be a cause effect relationship (obesity is a cause for diabetes) or the relationship between obesity (expressed by weight) and diabetes (expressed by blood glucose level). Alternatively, the purpose may be to test the null hypothesis (results are not because of chance), that is testing if the effect is only because of the input variables. The aim is to examine whether input (explanatory) variables relate to the effect (output or outcome) variables. Public health or medical research centers on an input to output relationship.
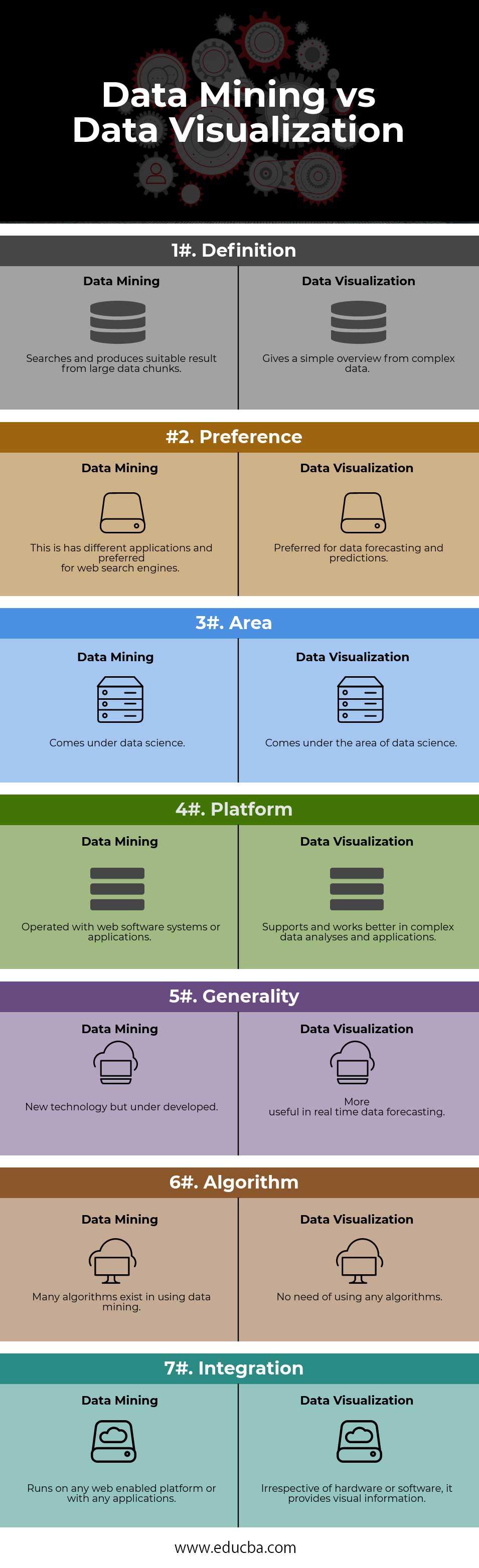
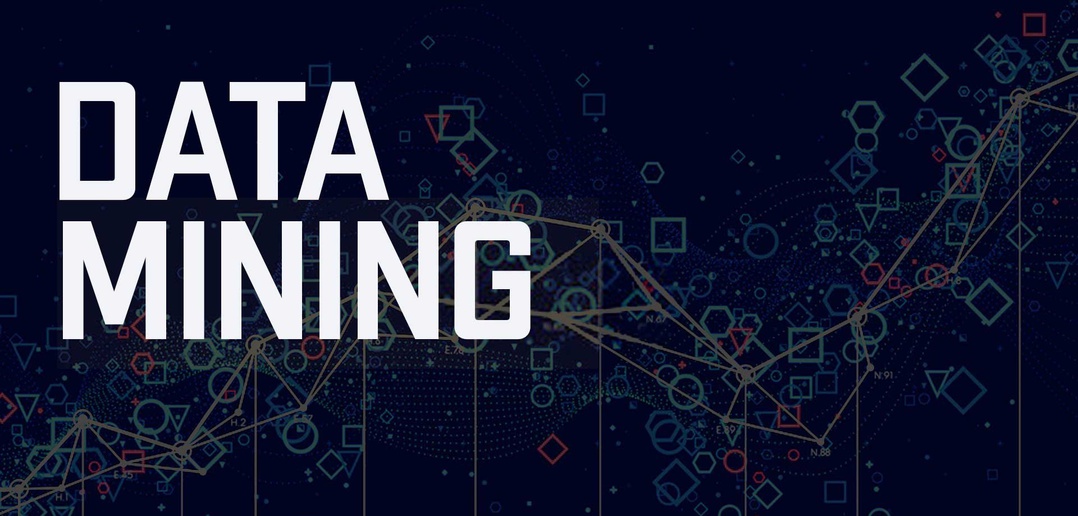
The aim of this essay is to provide a brief yet, a comprehensive review on the problem of multiple comparisons, and how data fishing risks public health studies’ outcomes. The problem of multiple comparisons is met with in many clinical trials, epidemiological studies, or public health studies, in which case, data fishing is a possibility.
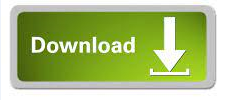